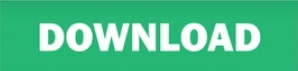
Hence, the completion suggester uses data structures that enable fast lookups but are costly to build and are stored in memory. The auto-complete functionality should be as fast as the user types to provide instant feedback relevant to what a user has already typed in. For the same reason mentioned above, it will increase the index storageĮlasticsearch provides a Completion Suggester as a native solution for auto-complete/search-as-you-type functionality.You can control which all keys to be returned using the source in while querying. Completion suggester will return the whole document as part of the suggest. In graphical user interfaces, users can typically press the tab key to accept a suggestion or the down arrow key to accept one of several. Completion suggester will not return the whole document as its just a suggester and doesn't work like full-text search which returns the whole document. Since the tokenizer breaks the text down into words on custom characters, it might increase the index time Autocomplete, or word completion, is a feature in which an application predicts the rest of a word a user is typing.Let’s assume I have an index with hotels like the example on the ElesticSearch website. However I’m struggling a little bit with permissions. It's better to use the same analyzer for both index and search I’m currently evaluating the Completion Suggester functionality in ElasticSearch to realize auto complete.

The query returns the documents that contain the words of a provided prefix text, in the same order as provided.Ī few points to be considered while implementing this approach: In this approach, we need to use the prefix query against the search field. Let's take a look at all these four approaches and see which approach is optimal and has a better implementation:
#Completion suggester elasticsearch how to#
How to Achieve Typeahead Search With ElasticsearchĮlasticsearch provides four different ways to achieve the typeahead search. This feature certainly helps the end users' experience while searching. If so, all the partially matched texts to the user are a way of providing hints when typing the text. Typeahead search, also known as autosuggest or autocomplete feature, is a way of filtering out the data by checking if the user input data is a subset of the data. These provide a better search experience and features like typeahead, fuzzy search, boosting the search results based on relevancy, similarity search, etc. There are many search engines available in the market today like Elasticsearch, Apache Solr, Azure Cognitive Search, etc.

This requires us to design and implement a search engine along with your golden source (RDBMS/NOSQL). Today, search is an important functionality in enterprise applications and end-users are obsessed with the experience of Google Search and expecting the application search also provides similar experiences.
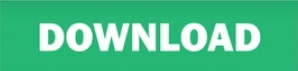